Planet-hunting with machine learning
- Min-Kai Lin
- Jul 24, 2020
- 2 min read
A Machine Learning model to infer planet masses from gaps observed in protoplanetary disks, Auddy, S., Lin, M.-K., ApJ, accepted
Observations of disks around young stars often show dust rings and gaps (Andrews et al., 2018; Long et al. 2018). The poster child for this is the disk around the star HL Tau, shown on the left. The origin of these structures is now one of the biggest mysteries in astronomy.
One popular explanation is that hidden planets carve gaps in the disk and solids become trapped at gap edges to form dust rings. Indeed, by comparing specialized simulations with observations, it is possible to deduce the masses of planets in these disks. However, with structured disks being discovered at an ever-increasing rate, we are well into the era of big data, and it is becoming less practical to develop customized computer models to interpret each observation.
In this newly accepted paper lead by Dr. Sayantan Auddy, Academia Sinica Postdoctoral Fellow, we propose a fast, future-proof model of planet gaps by taking advantage of machine learning. We develop DPNNet (Disk-Planet Neural Network) - an artificial deep neural network - to find hidden planets from gaps observed in protoplanetary disks.
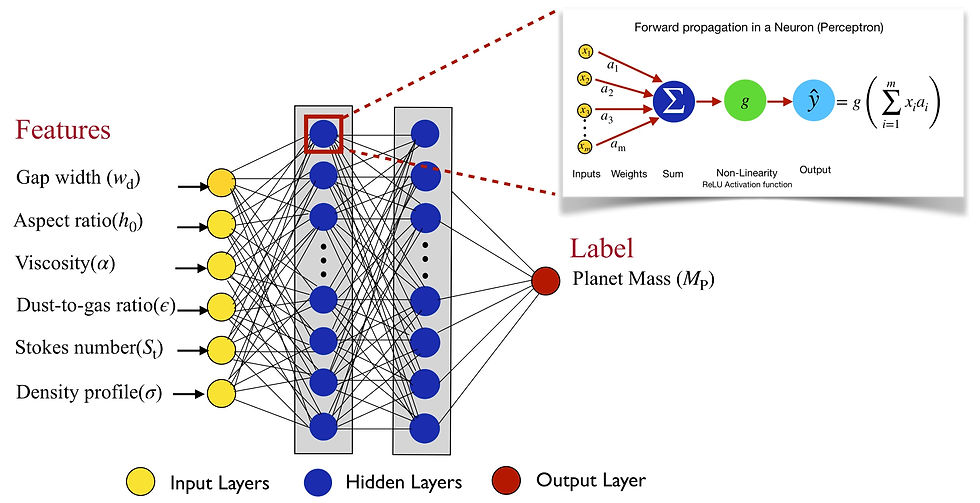
We ran ~1000 simulations of disk-planet interaction using the FARGO3D code to train DPNNet. This was made possible by running the code on Graphics Processing Units (GPUs) on the TAIWAINA-2 cluster hosted by the National Center for High-performance Computing in Taiwan.
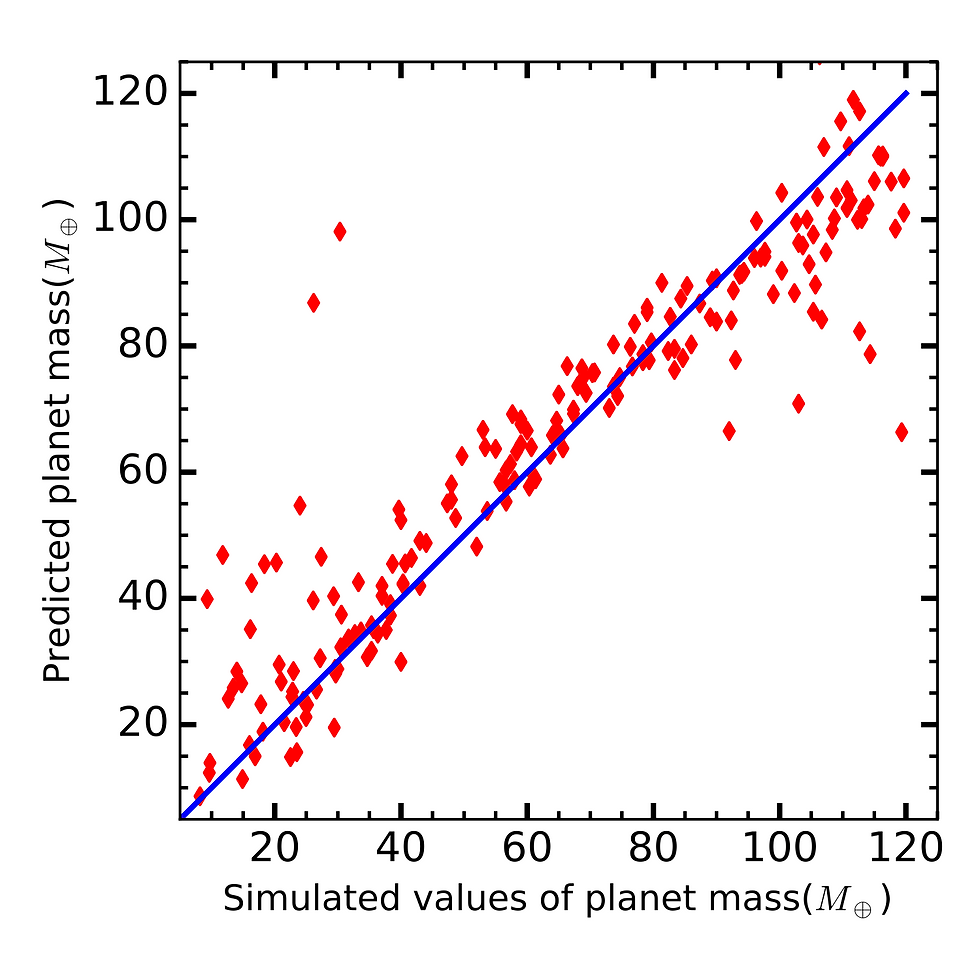
To test DPNNet, we use it to predict planet masses from gaps in simulations that the network has never seen. The result, shown on the right, is remarkable given the highly complex nature of disk-planet interaction. DPNNet is able to predict planet masses based on gap profiles with good accuracy.
We then applied DPNNet to the disk around HL Tau, finding that three planets with masses 0.25, 0.2, and 0.22 Jupiter masses can explain the observed gaps. These values are similar to those inferred from customized simulations (e.g. Dong et al., 2015). By contrast, once trained, DPNNet can be deployed to interpret observations without running simulations.
With further development, we hope DPNNet will allow disk modelers and observers to quickly infer the properties of hidden planets without performing sophisticated simulations. This, in turn, will help guide future observations in finding these new worlds.
Comments